Inhibition Potencies of Phytochemicals Derived from Sesame Against SARS-CoV-2 Main Protease: A Molecular Docking and Simulation Study
- 1Centre for Agricultural Bioinformatics (CABin), ICAR- Indian Agricultural Statistics Research Institute, New Delhi, India
- 2Division of Germplasm Evaluation, ICAR-National Bureau of Plant Genetic Resources, New Delhi, India
The ongoing COVID-19 pandemic, caused by SARS-CoV-2, has now spread across the nations with high mortality rates and multifaceted impact on human life. The proper treatment methods to overcome this contagious disease are still limited. The main protease enzyme (Mpro, also called 3CLpro) is essential for viral replication and has been considered as one of the potent drug targets for treating COVID-19. In this study, virtual screening was performed to find out the molecular interactions between 36 natural compounds derived from sesame and the Mpro of COVID-19. Four natural metabolites, namely, sesamin, sesaminol, sesamolin, and sesamolinol have been ranked as the top interacting molecules to Mpro based on the affinity of molecular docking. Moreover, stability of these four sesame-specific natural compounds has also been evaluated using molecular dynamics (MD) simulations for 200 nanoseconds. The molecular dynamics simulations and free energy calculations revealed that these compounds have stable and favorable energies, causing strong binding with Mpro. These screened natural metabolites also meet the essential conditions for drug likeness such as absorption, distribution, metabolism, and excretion (ADME) properties as well as Lipinski’s rule of five. Our finding suggests that these screened natural compounds may be evolved as promising therapeutics against COVID-19.
Introduction
The ongoing pandemic eruption due to the worldwide spread of coronavirus disease (COVID-19) is caused by the novel virus strain severe acute respiratory syndrome coronavirus-2 (SARS-CoV-2; previously named 2019-nCoV) (Wu et al., 2020b). This viral disease is an unprecedented global public health care threat (Jamwal et al., 2020). The first case of COVID-19 disease was originated from Wuhan, Hubei Province, China, and quickly spread across 219 countries and territories around the world with high mortality rates in immunocompromised patients (Enayatkhani et al., 2020; Mackenzie and Smith, 2020; Xu et al., 2020). Based on the recommendations of the Emergency Committee, the World Health Organization (WHO) has declared this respiratory infectious disease as a Public Health Emergency of International Concern (PHEIC) on 30 January, 2020 and a pandemic on 11 March, 2020 (Shi et al., 2020; Yu et al., 2020). As on 10 July 2021, this contagious disease had led to more than 185,291,530 confirmed cases and 4,010,834 fatalities (https://covid19.who.int/), with the number of cases increasing abruptly across the globe. At present, India is fighting hard against the second wave of COVID-19. The ongoing pandemic has now initiated taking a toll on India’s economy. A large population of India is facing disproportionately higher rates of COVID-19 infection, morbidity, and mortality. As of 10 July 2021, the total COVID-19 caseload has now soared to 30,752,950 with 405,939 deaths (https://covid19.who.int/table). India is the most severely affected Asian country. The ongoing pandemic has been considered more dreadful than the previous global outbreaks, namely, SARS-CoV (2002–2003) and Middle East respiratory syndrome (MERS) (2012–present) (de Wit et al., 2016; Gupta et al., 2020; Wang et al., 2020b; Wu and McGoogan, 2020; Yuan et al., 2020). Based on previous investigations, the fatality rate of SARS-CoV and MERS has been calculated as 10 and 35%, respectively (Lee et al., 2004; Cheng et al., 2007). It has been well-reported that COVID-19 affects the lower respiratory tract of the body, which causes pneumonia and affects the gastrointestinal system, kidney, heart, and central nervous system. Fever, cough, diarrhea, and tiredness have been considered the most common symptoms (Chen et al., 2020a; Tang et al., 2020), while aches and pains, sore throat, conjunctivitis, headache, loss of taste or smell, a rash on skin, or discoloration of fingers or toes are the less common symptoms of this infectious disease (Backer et al., 2020; Rothe et al., 2020; Russell et al., 2020; Verdoni et al., 2020; Yu and Yu, 2020).
The coronaviruses have been recognized as a large enveloped positive-sense single-strand RNA viruses from Nidovirales (order) of the Coronaviridae family and subfamily Coronavirinae (Raj et al., 2021; Shamsi et al., 2021). This subfamily is classified into four genera including alpha-, beta-, gamma-, and deltacoronavirus (α-, β-, γ-, and δ-CoV) based on evolutionary methods (Hulswit et al., 2016). In view of previous reports, coronaviruses have been considered as highly evolving viruses, with a high rate of mutation and genomic recombination (Chen et al., 2020b). In the past, six species of human coronavirus associated with different respiratory tract diseases have been reported, which include HCoV-NL63, HCoV-229E, HCoV-OC34, HCoV-HKU1, SARS-CoV, and MERS-CoV (Arden et al., 2005; Woo et al., 2005; Su et al., 2016). The novel strain SARS-CoV-2 has been characterized as the seventh strain of the human coronavirus. Based on the significant nucleotide sequence similarity with SARS and MERS coronaviruses, the International Committee on Taxonomy of Viruses (ICTV) coined the nomenclature of SARS-COV-2 (Hasan et al., 2020). The ICTV taxonomically placed the SARS-COV-2 in the genus Betacoronavirus (Helmy et al., 2020; Wang et al., 2020b).
The genome size of SARS-CoV-2 is ∼29.9 kb (29,903 nucleotides) (Wu et al., 2020a). The first whole-genome sequencing data for SARS-CoV-2 (∼30 kb) were submitted to the Genbank with the accession number MN908947 and isolated from Wuhan (Wu et al., 2020a). The genome of SARS-CoV-2 encodes approximately 13–15 open reading frames (ORFs) which are flanked by 5′ and 3′ UTRs (Chen et al., 2020b; Elfiky and Azzam, 2020; Gordon et al., 2020). These ORFs constitute a replicase assembly during the replication process of the central dogma of molecular biology and encode 27 distinct structural and non-structural proteins (NSPs) (Liu et al., 2021; Shamsi et al., 2021). The 5′ end of the SARS-CoV-2 genome encodes 16 NSPs (Nsp1-16) and constitutes the replicase/transcriptase complex (RTC). These 16 proteins are conserved in all SARS viruses and play a critical role in a set of biological processes such as viral replication, assembly, and immune response modulation (Shamsi et al., 2021). The 3′ end of the viral genome encodes four conical structural proteins including E (envelope protein), M (membrane protein), N (nucleocapsid protein), and S (spike protein), and nine putative accessory factors. The main protease enzyme (Mpro also called 3CLpro) is essential for viral replication and has been considered as one of the potent drug targets for treating COVID-19 (Joshi et al., 2020; Khan et al., 2020; Kumar et al., 2020; Pant et al., 2020; Wu et al., 2020a; Zhang et al., 2020). In cooperation with other components, this important enzyme also helps in the transcription of the viral RNA. Mpro is a key enzyme that exclusively cleaves the polyproteins (pp1a and pp1ab) which is essential for the assembly of virus drugs (Jin et al., 2020). The molecular mass of Mpro is 33,797 Da with length of 306 amino acid residues and structurally possesses the three functional domains, namely, domain I (8–101 residues), domain II (102–184 residues), and domain III (201–306 residues) (Jin et al., 2020; Khan et al., 2020). Among them, domains I and II have an antiparallel β-barrel structure, while domain III represents a group of five α-helices organized as a large antiparallel cluster. Domain III is connected to domain II by a 15-residue-long loop region (185–200 residues). The active site is composed of a catalytic dyad having Cys145 and His41 residues (Khan et al., 2020). The functional role of Mpro in the viral replication highlights its importance that can be used to identify the potential drug therapeutics against COVID-19 (Ullrich and Nitsche, 2020). Solved crystal structures of Mpro provide a platform to develop and design the antiviral drugs to combat COVID-19 (Jin et al., 2020; Zhang et al., 2020). In response to the COVID-19 outbreak, several studies have been performed using integrated bioinformatics and molecular modeling approaches for the screening of novel natural metabolites as potential drug targets against Mpro (Chikhale et al., 2020a; Kumar et al., 2020; Maurya and Sharma, 2020; Rout et al., 2020; Tripathi et al., 2020; Mishra et al., 2021; Romeo et al., 2021; Tock et al., 2021). But no effective method has been developed yet to prevent and treat the COVID-19 disease in a significant manner. In addition to the aforementioned approaches, several other viral protease inhibitors like remdesivir, hydroxychloroquine, chloroquine, lopinavir, ritonavir, oseltamivir, and fapilavir have been explored as repurposed drugs for COVID-19 treatment (Chang et al., 2016; Chang et al., 2020; Contini, 2020; Das et al., 2020; Elfiky, 2020; Gonzalez–Paz et al., 2020; Islam et al., 2020; Khan et al., 2020; Sinha et al., 2020; Wahedi et al., 2020; Abdelli et al., 2021). The antimalarial drug named as chloroquine has been proposed as the potential inhibitor of Mpro activity (Ou et al., 2021). In a recent follow-up study, Pathak et al. (2021) explored the potential of rifampicin and letermovir as repurposed drug candidates against COVID-19. On the contrary, several studies reported the severe adverse effects of these repurposed drugs in different countries (Sultana et al., 2020; Wang et al., 2020a). Therefore, it is imperative to discover natural compound–based drug targets that could serve as potential inhibitors of different SARS-CoV-2 proteins and aid in controlling viral replication to enhance efficacy in COVID-19 treatment.
Sesame (Sesamum indicum L.) is an herbaceous annual plant cultivated for its edible seed, oil, and flavorsome value, belonging to the order Tubiflorae, family Pedaliaceae with many common names including gingelly, til, and benne seed (Bhat et al., 2014; Pathak et al., 2019). This oil crop is regarded as “queen of oilseeds” because of its property of resistance to oxidation and rancidity (Dalibalta et al., 2020). Sesame is widely cultivated in the tropical parts of Africa and Asia, India being one of the major producers with Myanmar, China, and Sudan (Majdalawieh et al., 2017). A plethora of nutrients including proteins, carbohydrates, antioxidants, lignans, tocopherols, phytates, phytosterols, and polyunsaturated fatty acids are exclusively found in sesame (Nagendra Prasad et al., 2012; Kumar et al., 2018; Pathak et al., 2019). These bioactive compounds possess certain medicinal properties like hepatoprotective, hypoglycemic, antihypertensive, anti-estrogenic, and anticancer (Kumar and Singh, 2014; Majdalawieh et al., 2017). Active ingredients of sesame have also been investigated as potential inhibitors of Parkinson’s disease (PD) (Kappo et al., 2016). There are very few reports available for the screening of sesame-derived compounds against main protease of COVID-19. So far, only one compound of sesame, namely, sesamin has been well-explored against COVID-19 using in silico approach. Kodchakorn et al. (2020) investigated the potential of sesamin along with other herbal medicines (andrographolide, anthocyanin-b-D-glucoside, capsaicin, curcumin, cyanidin, cyanidin-3-O-glucoside, and hesperidin) against the Mpro of SARS-CoV-2 using molecular docking. Docking complexes of these nutraceuticals with Mpro were further validated for their atomic stability using molecular dynamics (MD) simulations on 50 ns, and suggested that the screened compounds may be considered for coprotection and treatment against COVID-19. In a recent study, Pandey and Verma (2020) also studied the potential of sesamin and four other dietary components (galangin, ellagic acid, capsaicin, and epicatechin) as structural inhibitors of SARS-CoV-2 Mpro using the molecular docking approach. In a very recent study, Allam et al. (2021) reported seven sesame-derived natural compounds (sesamin, sesamolin, pinoresinol, hydroxymatairesinol, spicatolignan, ferulic acid, and vanillic acid) as potential inhibitors against three proteins of SARS-CoV-2 including Mpro, papain-like protease (PLpro), and the RNA-dependent RNA polymerase (RdRp) using the molecular docking analysis followed by MD simulations on 50 ns for representative complexes. However, there is no significant evidence of docking results evaluation available for MD simulations on high nanosecond scale (up to 200 ns) to understand the inhibitory mechanism of all sesame-derived compounds against the SARS-CoV-2 proteins. Despite the medicinal importance of sesame, all bioactive molecules derived from this important medicinal plant have not been well-explored in a significant manner yet for the treatment of COVID-19. With the fruitful utilization of molecular modeling methods including molecular docking and MD simulations, sesame-derived bioactive compounds may be utilized to design the alternative natural compound–based effective therapeutics against COVID-19.
Keeping this in view, in the present study, we have undertaken a thorough attempt to investigate the inhibition potencies of 36 phytochemicals from sesame against Mpro of SARS-CoV-2 using the molecular docking approach. Four natural metabolites, namely, sesamin, sesaminol, sesamolin, and sesamolinol, were further subjected to conformational stability using MD simulations followed by free energy calculations. The knowledge generated in the current study encourages and suggests that the sesame-derived phytochemicals have enough potential of being effective in treatment of COVID-19.
Materials and Methods
A flowchart depicting the pipeline involved in the identification of interaction between sesame-derived bioactive molecules and Mpro is presented in Figure 1.
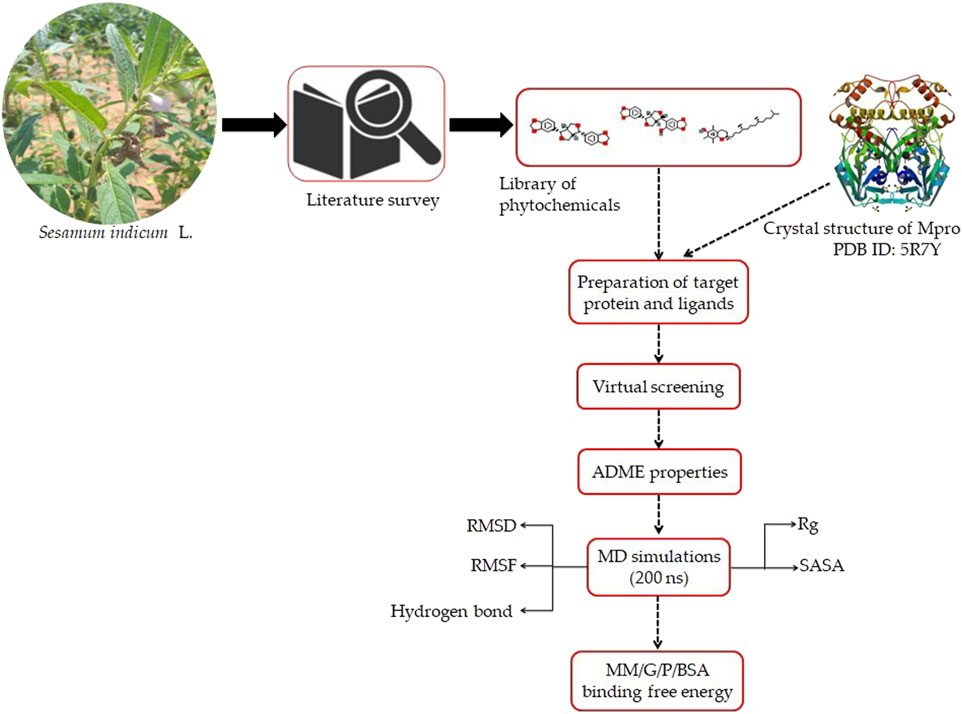
FIGURE 1. Representation of pipeline utilized in the present study to identify the inhibitors of Mpro of SARS-CoV-2 using an extensive molecular modeling approach.
Ligand Selection
An extensive literature survey was conducted to prepare a library of sesame-derived natural compounds reported with therapeutic potential. Chemical structures of 36 phytochemicals (Supplementary Table S1) were obtained from the PubChem database (Kim et al., 2020) in a Spatial Data File (SDF) format. All these molecules were optimized prior to molecular docking using a set of AutoDock tools (Morris et al., 2009). Each and every molecule embedded in thse library was prepared with the addition of polar hydrogens and Gasteiger charges calculation. For the docking purpose, the molecules were saved in a pdbqt format using PyRx Open Babble tools (O'Boyle et al., 2011).
Preparation of Receptor
The crystal structure of the Mpro of SARS-CoV-2 in a complex with Z45617795 (PDB ID: 5R7Y) was attained from the RCSB-Protein Data Bank (Berman, 2000; Burley et al., 2018) for docking purposes. This protein crystal structure was solved by the PanDDA analysis group (https://www.rcsb.org/structure/5R7Y). Preprocessing of the Mpro of SARS-CoV-2 was carried out by removing water atoms and heteroatoms, and adding polar hydrogen atoms and Kollman charges on it using AutoDockTools version 1.5.6. Swiss-pdb Viewer (Guex and Peitsch, 1997) was employed to structure optimization and energy minimization. The clean geometry module available in the Discovery Studio platform was utilized for the side chain correction.
Virtual Screening Based on Molecular Docking
In a search for a drug against COVID-19, we performed a site-specific docking screen for the Mpro of SARS-CoV-2 against the prepared library of sesame-derived natural compounds containing 36 compounds. AutoDock Vina program was employed for virtual screening. The grid box was created with the size of 70 Å × 70 Å × 70 Å, with a total of 50 genetic run. For the purpose of docking, amino acid residues such as Thr24, Thr26, Asn119, Phe140, Gly143, Cys145, His163, His164, Glu166, Gln189, and Thr190 were considered as active sites, as earlier reported by Khan et al. (2020) and Kumar et al. (2020). Other parameters were set as default while docking process. The carmofur (CID_2577) compound was selected as the positive control (Jin et al., 2020) for docking process. After docking, the top ranked compounds (based on docking score, number of hydrogen bonds, and specificity) (Table1) were chosen and visually inspected using PyMol and Discovery Studio (DeLano, 2002).
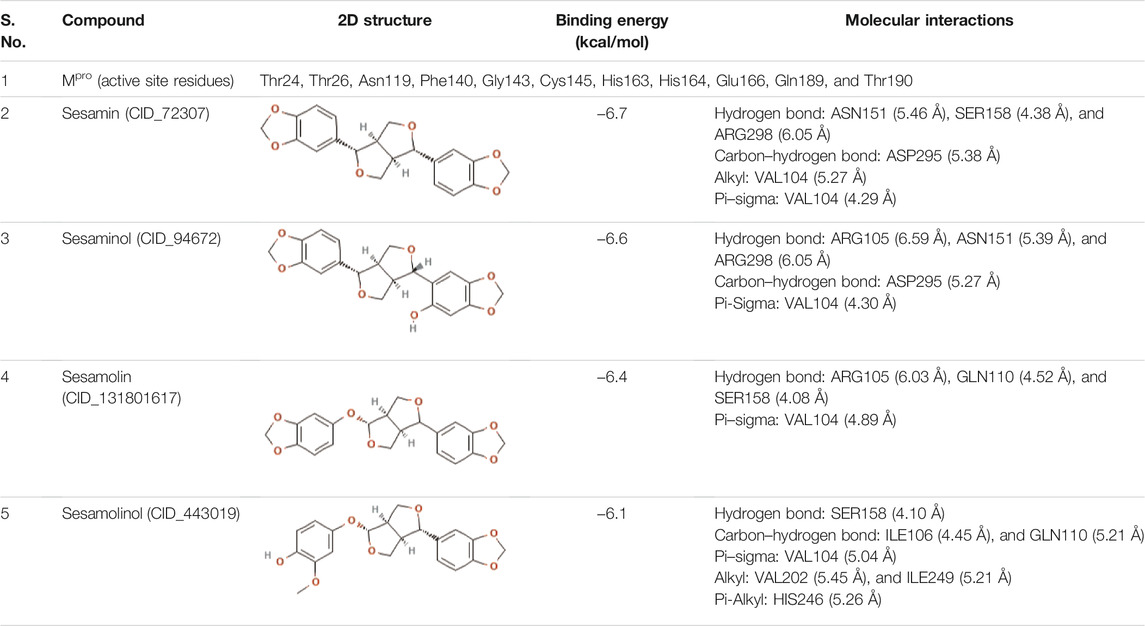
TABLE 1. List of top four natural compounds shortlisted based on binding energy score as a result of virtual screening.
Drug-Likeness and Absorption, Distribution, Metabolism, and Excretion Profiling
The automated Swiss ADME server (Daina et al., 2017) was employed to calculate the drug-likeness attributes of screened molecules. Different molecular properties such as molecular weight, number of hydrogen bond accepters, number of hydrogen bond donors, number of rotatable bonds, molar refractivity, bioavailability score, synthetic accessibility, TPSA, and solubility were calculated with utilizing Lipinski’s rule of five (Lipinski, 2004) and Veber’s rule (Veber et al., 2002).
Molecular Dynamics Simulations
In order to assess the stabilities of docking conformation complexes of the four bioactive compounds sesamin, sesaminol, sesamolin, and sesamolinol with SARS-CoV-2 Mpro, MD simulations were performed using GROMOS9643a1 force field embedded in GROMACS 5.1.1 package installed on Linux-based workstation (Abraham et al., 2015; Kutzner et al., 2019). For the MD simulations, we followed the protocol previously described by Gajula et al. (2016) and Jee et al. (2017). The automatic PRODRG server was employed to prepare the topology files of ligand molecules (Schüttelkopf and van Aalten, 2004). The docking complexes were solvated in a dodecahedron box. In order to make the whole system neutral, the appropriate Na+ ions were added to the system. The steepest descent algorithm was applied to perform the energy minimization of the prepared system with 50,000 iteration steps and cutoff up to 1,000 kjmol−1 with a primary goal of reducing the steric clashes during simulations. The long-range electrostatic interactions were calculated by using particle mesh Ewald (PME) truncation method (Abraham and Gready, 2011). Prior to a production run, the process of equilibrium was completed in two phases. In the first phase, equilibration was established with a constant number of particles, volume, and temperature (NVT), with each step 2 femtosecond (fs). The second phase was performed with a constant number of particles, pressure, and temperature NPT, with the ensemble at 300 K. After determining the coordinates, LINCS algorithm was considered to constrain the covalent bonds involving hydrogen atoms (Hess et al., 1997; Hess, 2007). Temperature was regulated inside the box using V-rescale, a popular Berendsen temperature coupling method. Finally, a production run of 200 ns was run with each step of 2 fs.
Trajectory Analysis
After the successful completion of MD simulations, trajectories were analyzed using a set of tools implemented in GROMACS package. The gRMS tool of GROMACS was utilized to calculate the root-mean-square deviation (RMSD) variation in protein backbone, while the overall root-mean-square fluctuation (RMSF) in the atomic positions of protein C backbone was generated by using the grmsf module. The gyrate, gmxsasa, and g h bond tools were employed to estimate the radius of gyration (Rg), solvent accessible surface area (SASA), and hydrogen bonds, respectively.
Molecular Mechanic/Poisson–Boltzmann Surface Area Binding Free Energy Calculations
The Molecular Mechanic/Poisson–Boltzmann Surface Area (MM/PBSA) was performed on g mmpbsa script program to calculate the binding free energy of interactions between the docking complexes (Kumari et al., 2014; Aldeghi et al., 2017). After the simulation of docking complexes, all the trajectories of 200 ns were used for MM/PBSA-based binding free energy analysis. The major energy components such as binding energy (kJ/mol), van der Waals energy (DEvdW), electrostatic energy, polar solvation energy, and SASA energy all together contributed to calculate the MM/PBSA relative binding affinity. The MM/PBSA method–based binding free energy of the protein–ligand systems were calculated using the following equation:
where Gcomplex represents the total free energy of the docking complex, and Gprotein and Gligand depict the total free energies of the isolated protein and ligand in the solvent, respectively.
Results and Discussion
Molecular Docking
Molecular docking is one of the most applied methods in the process of computer-aided drug design (CADD) to identify potential inhibitors against various pathogens. With this revolutionary method, an immense amount of energy, time, and costs of the drug discovery process can be saved to screen the large drug libraries for the discovery of potential drug compounds (Wadood et al., 2013; Yu and MacKerell, 2016). There is no effective cure for COVID-19 so far; therefore, identification of potential drug compounds is required on an urgent basis. In the present study, we screened an in-house library of sesame-derived bioactive molecules against Mpro of SARS-CoV-2 using a molecular docking approach. In total, 36 natural compounds (Supplementary Table S1) were docked into the binding pocket of Mpro. The docking results demonstrated that out of 36 selected natural compounds used in the present study, four bioactive molecules, namely, sesamin, sesaminol, sesamolin, and sesamolinol were found to have a higher binding energy of −6.7, −6.6, −6.4, and −6.1 kcal/mol−1, respectively, than the positive control compound carmofur whose binding energy was determined to be −5.2 kcal/mol−1. These four natural compounds (sesamin, sesaminol, sesamolin, and sesamolinol) ranked as top interacting with Mpro based on the affinity of molecular docking, number of hydrogen bonds and compound specificity.
The 2D structures, binding score, and details of interactions of the top four screened compounds are displayed in Table 1. Docking complexes of these natural metabolites with Mpro have been considered for further evaluation using MD simulations and MM/PBSA energy calculations. Discovery studio and PyMOL programs were employed to prepare the two- and three-dimensional plots of molecular interaction networks, respectively. After visualizing the 2D and 3D interaction plots, it was observed that the sesamin compound formed hydrogen bonds with three residues, namely, Asn151 (5.46 Å), Ser158 (4.38 Å), and Arg298 (6.05 Å). This compound was found to have one carbon–hydrogen (C–H) bond with Asp295 (5.38 Å), alkyl bond with Val104 (5.27 Å), and Pi–sigma bond with Val104 (4.29 Å) residue. It also manifests van der Waals (VdW) interaction with six residues including Arg105, Ile106, Gln110, Thr111, Thr292, and Phe294 (Figure 2).
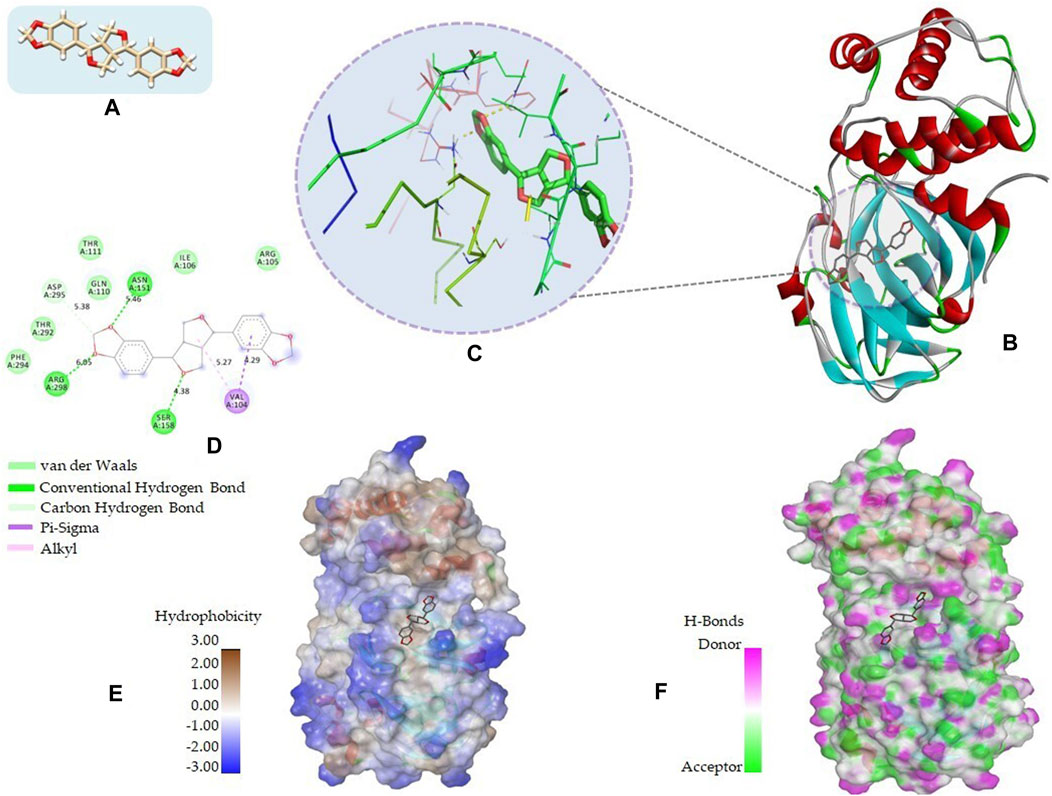
FIGURE 2. 2D and 3D representation of molecular interaction between the Mpro of COVID-19 (PDB ID: 5R7Y) and sesamin compound (CID_72307): (A) 3D structure presentation of sesamin; (B) Molecular docking complex of a crystal structure of Mpro with sesamin molecule, docked using AutoDock implemented in PyRx package; (C) close view of pocket with sesamin structure in the stick model colored by atom types, yellow dashed lines represent the hydrogen bond networks; (D) 2D representation of different types of interactions with sesamin including van der Waals, conventional hydrogen bond, carbon hydrogen bond, Pi–sigma, and alkyl; (E) hydrophobicity surface representation of the overall structure of Mpro in complex with Sesamin; and (F) pocket view of sesamin binding with Mpro and the representation of residues involved in hydrogen bond donor acceptor. The docking complex of Mpro with sesamin was rendered in different CPK using UCSF Chimera, Discovery Studio, and PyMol.
In the case of sesaminol, three residues, namely, Arg105 (6.59 Å), Asn151 (5.39 Å), and Arg298 (6.05 Å), formed the hydrogen bonds. Residues Asp295 (5.27 Å) and Val104 (4.30 Å) interacted via C–H bond and Pi–sigma, respectively. Five residues including Ile106, Gln110, Thr111, Thr292, and Phe294 manifest VdW interaction (Figure 3A). As shown in Figure 3B, sesamolin molecule exhibits the hydrogen bond with three residues, namely, Arg105 (6.03 Å), Gln110 (4.52 Å), and Ser158 (4.08 Å), and one Pi–sigma with Val104 (4.89 Å). VdW interaction with residues Phe8, Lys102, Phe103, Thr111, Asn151, Thr292, and Phe294 was also formed. In the sesamolinol molecule, one residue Ser158 (4.10 Å) formed hydrogen bond (Figure 3C). Several other residues formed other types of molecular interactions such as Ile106 (4.45 Å), Gln110 (5.21 Å) (C–H bond), Val104 (5.04 Å) (Pi–sigma), Val202 (5.45 Å), Ile249 (5.21 Å) (alkyl), His246 (5.26 Å) (Pi–alkyl), and residues Arg105, Gln107, Asn151, Asp245 demonstrated VdW interactions.
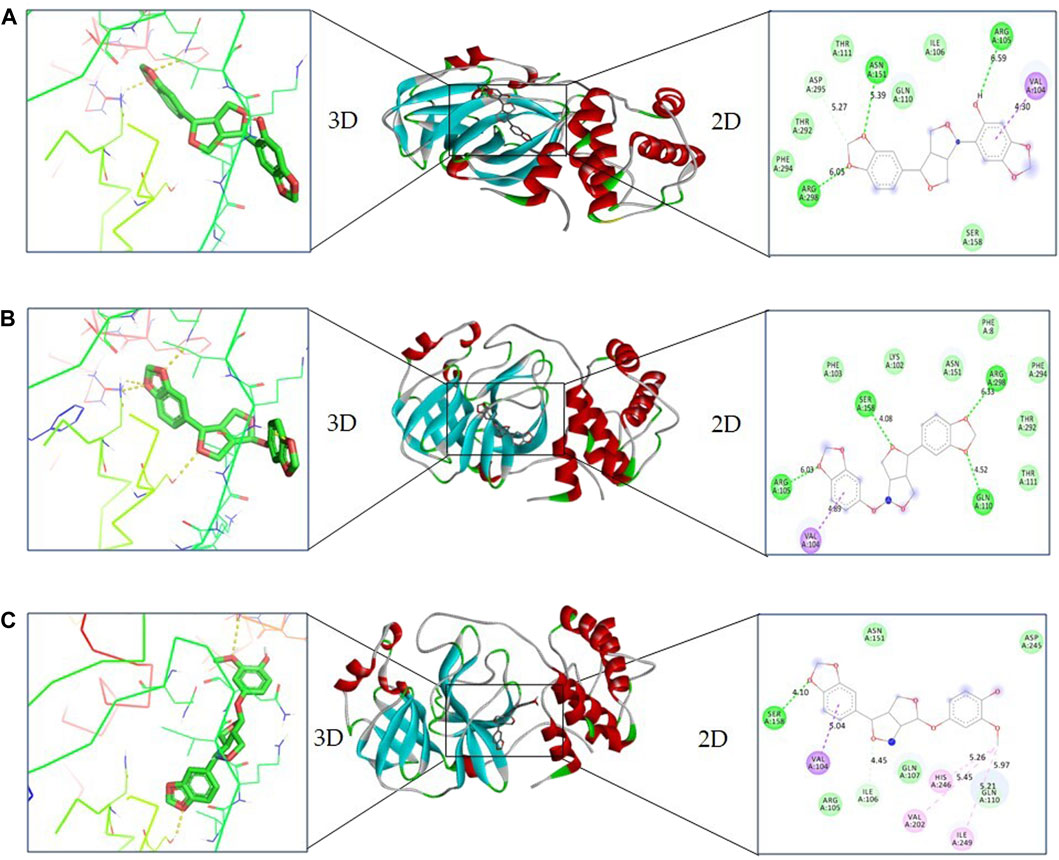
FIGURE 3. 2D and 3D representation of docking complexes: (A) Mpro and sesaminol complex; (B) Mpro and sesamolin complex; (C) Mpro and sesamolinol complex visualized using UCSF Chimera and Discovery Studio.
Consistent with previous studies which reported the potential inhibitors of Mpro (Park et al., 2015; Aanouz et al., 2020; Bello et al., 2020; Chikhale et al., 2020b; Krupanidhi et al., 2020; Matveeva et al., 2020; Muhammad et al., 2020; Tripathi et al., 2020; Mitra et al., 2021; Prasanth et al., 2021; Varadharajan et al., 2021), in our study, screened four compounds (sesamin, sesaminol, sesamolin, and sesamolinol) were found to be tightly fit into the binding pocket of the Mpro of COVID-19. In previous studies, the potential of herb-derived natural compounds have been explored to inhibit the Mpro of COVID-19 using integrated bioinformatics and molecular modeling approaches (Kumar et al., 2020; Suravajhala et al., 2020; Gunda et al., 2021; Mishra et al., 2021). Three natural metabolites, namely, ursolic acid, carvacrol, and oleanolic acid have been reported as the potential inhibitors of Mpro of COVID-19. The molecular docking study of ursolic acid, carvacrol, and oleanolic acid with the Mpro protein demonstrated the binding energy of −5.9, −4.0, and −6.0 kcal/mol, respectively (Kumar et al., 2020). The ursolic acid formed a strong hydrogen bond with Ser46 residues, while the docking study of carvacrol and oleanolic acid with the Mpro protein exhibits hydrogen bonding with Gly143 and Gln189 residues of the active site, respectively. In a recent study, Gunda et al. (2021) proposed the natural xanthone compounds as promising drug inhibitors against the Mpro of COVID-19 based on their significant antiviral power, which is well-documented in literatures. In a recent follow-up study, Mishra et al. (2021) explored a set of natural compounds to investigate their binding potential to the Mpro of COVID-19. Based on the docking and MD simulations, the four natural compounds, namely, amentoflavone, guggulsterone, puerarin, and piperine have been reported as antiviral compounds against the Mpro of COVID-19. The binding affinity of these natural metabolites with Mpro protein confirms the results of the present study.
Several compounds of sesame possess the natural antibacterial, antifungal, antiviral, and anti-inflammatory properties, and lignans such as sesamin, sesaminol, sesamolin, and sesamolinol are good examples (Uncu et al., 2015; Dravie et al., 2020). Sesamin is exclusively found in the sesame plant, and its antioxidant, antibacterial, antiviral, and antifungal activities are well-reported. Kodchakorn et al. (2020) identified that sesamin interacts with the Mpro of SARS-CoV-2 and affects the thermal stability of Mpro using in silico methods, providing evidence for sesamin as a structural inhibitor against the Mpro of SARS-CoV-2. Other studies also indicated that the sesamin compound might interact with amino acid residues Ser144, Cys145, Gln189, and Gln192 and showed significant interactions with effective residues His41, Met49, and Met165 of the Mpro of COVID-19 (Pandey and Verma, 2020). In a follow-up study, Allam et al. (2021) explored the sesamin and sesamolin compounds along with other natural compounds against Mpro, PLpro, and RdRp proteins. Sesamin was found to be interacted with Mpro with three residues including Gln189, Thr190, and His41, while the sesamolin molecule was reported to interact with two amino acid residues, namely, Gln189 and Thr190. Our results may support the previous findings on the inhibitory effect of sesamin and sesamolin against the Mpro of COVID-19. Previous reports demonstrated the docking results only for few compounds including sesamin and sesamolin but did not consider all compounds of sesame reported in the literature, which have significant medicinal properties as well. In the present study, we explored the potential of 36 sesame-derived natural compounds against the Mpro of COVID-19, and based on the docking results, the four natural compounds were selected, namely, sesamin, sesaminol, sesamolin, and sesamolinol for further evaluation using MD simulations on 200 ns. The previous studies lack the evidence of docking results evaluated using MD simulations on high ns scale. The screened natural compounds based on the present study were also well-studied for their central role in different biological activities. Several in vitro and in vivo studies illustrate the neuroprotective role of sesamin against cerebral ischemia (Chung et al., 2010; Dar and Arumugam, 2013). Also, this major lignin compound has demonstrated other biological activities such as antihypertensive, atherosclerosis, thrombosis, antidiabetic, anticancer, cardiovascular, and anti-inflammatory (Kumar et al., 2018; Dalibalta et al., 2020). Of note, sesamin has been previously shown to be effective against swine flu (influenza type A H1N1) through in silico and in vitro studies (Fanhchaksai et al., 2015). This compound was established as a novel inhibitor of pro-inflammatory cytokines, IL-1β and TNF-α. Sesaminol is one type of sesame lignan compound commonly found in sesame seeds and well known for its strong antioxidant and anticancer properties (Miyahara et al., 2001; Watanabe et al., 2017). Using in vitro and in vivo models, Kaji et al. (2020) reported the preventive effect of sesaminol on a neurodegenerative disease named as Parkinson’s disease (PD). Sesamolin, the second major lignan, found in sesame oil has been regarded as a natural therapeutic agent because of its various therapeutic properties (Michailidis et al., 2019). Free radical scavenging activity of sesamolin provides protection to neuronal hypoxia (Park et al., 2010). Sesamolinol has also been considered the important lignin compound due to its various biological activities (Grougnet et al., 2011). The present study reported bioactive molecules (sesaminol, sesamolin, and sesamolinol) which are established as potential inhibitors of Mpro having enough bibliographical research support.
Evaluation of Drug Likeness
Prior to conducting MD simulation analysis, we evaluated the pharmacokinetic properties of the screened compounds of sesame. The ADME results of the shortlisted molecules calculated using SWISSADME server are shown in Table 2. Sesamin, sesaminol, sesamolin, and sesamolinol have the following molecular weights, respectively: 354.35, 370.35, 370.35, and 372.37 g/mol; these four natural compounds have a molecular weight ≤500 g/mol, which indicated that these screened natural compounds may easily be transported, diffused, and absorbed by the body (Lipinski et al., 2001; Lipinski, 2004).The LogP values of sesamin, sesaminol, sesamolin, and sesamolinol molecules were found to have 2.79, 2.37, 2.74, and 2.56, respectively, which are in accordance with Lipinski’s rule of five. For these four compounds, the number of hydrogen bond donors was less than five, which meets the criteria of ADME as the number of H bond donors should be ≤5. The ADME analysis revealed that sesamin, sesaminol, sesamolin, and sesamolinol molecules present the following values of the topological polar surface (TPSA): 55.38, 75.61, 64.61, and 75.61 Å2. The range of lower TPSA values represents the acceptable results, as described by Ahuja et al. (2021) and Singh et al. (2021) in previous studies. It has been noted that the natural compounds derived from sesame are better behaved than the co-crystallized molecule. These screened molecules also validate Veber’s rule which state the oral bioavailability of drug-like compounds. These four metabolites, namely, sesamin, sesaminol, sesamolin, and sesamolinol have the molar refractivity values 90, 92.02, 91.52, and 93.98, respectively; these compounds also present the scores of the synthetic accessibility (SA): 4.12, 4.31, 4.43, and 4.50, respectively. SA is one of the important parameters of synthesis during the process of drug designing (Ertl and Schuffenhauer, 2009). The predicted SA score of these screened compounds was <10, which suggested that these compounds can be easily synthesized. Taken together, the drug-likeness analysis indicated that these sesame-derived natural metabolites possess favorable pharmacokinetic properties, and thus can be considered drug-like molecules.
Conformation of Stability of Docking Complexes for Natural Compounds and SARS-CoV-2 Mpro by Molecular Dynamics Simulations
In order to determine the structural stability of docking complexes, MD simulations were run with the most stable docked models on 200 ns. Based on docking scores, hydrogen bonds, and compound specificity, four docking complexes, namely, sesamin, sesaminol, sesamolin, and sesamolinol were subjected to MD simulations. High binding energy scores of docking complexes allowed for the estimation of the amino acid residue interactions over time. The RMSD, RMSF, SASA, and Rg plots were calculated to evaluate the stability of simulated systems.
Root-Mean-Square Deviation
The RMSD is a most commonly used quantitative method to evaluate the stability of the docking complexes and measures the conformational stability perturbations within the protein backbone during MD simulations on different nanosecond scales (Sargsyan et al., 2017). In order to investigate the stability of the ligand molecules to the protein, all the ligand and backbone RMSDs were graphically measured. As evident from Figure 4A, the protein backbone of Mpro showed constant stability throughout the simulation with a range between 0.37 and 0.47 nm. The average RMSD values for complexes with sesamin, sesaminol, sesamolin, and sesamolinol were ∼0.37, ∼ 0.38, ∼ 0.31, and ∼0.38 nm, respectively. Likewise, the control (blue) element also showed the average value of RMSD to be around 0.47 nm. The complex with sesaminol (yellow) and sesamolinol (cyan) displayed higher simulation trajectory after ∼50 ns than the complex with sesamin (red) and sesamolin (green). The compound sesamolin has shown two fluctuations throughout the simulations on 200 ns time scale. The first stable conformation was noted between 25 and 100 ns, and the second stable conformation was found between 110 and 200 ns. The RMSD constant was at ∼0.25, and a large fluctuation was observed between 10 and 25 ns. However, there was no significant effect of this fluctuation was found on the protein structure. Sesamolinol showed slight changes in the starting period of simulation between 2 and 25 ns. After 25 ns, sesamolinol was found to be constant at ∼0.35 throughout the simulations. It may be because of the binding region size and loop presence at the pocket site. All the four ligand molecules shared the almost similar trend of stability and RMSD values with small conformational changes. As depicted in Figure 4B, the calculated ligand RMSD plot is the conformation of the measured protein backbone; RMSD plot shows the stability of target compounds throughout the simulation with fluctuation in sesamolinol at starting point between 5 and 15 ns on ∼0.50 nm. In the same plot, the sesamolin compound also showed the fluctuation between 160 and 170 ns on ∼0.25 nm. Based on the minimal fluctuations and low difference in values depicted in the protein backbone and ligand RMSD plots, it can be predicted that protein–ligand complexes were stable and comparable to solved structures. The docked pose of our ligands is fixed in the active region, same as the crystal structure ligand Z45617795, which is quite acceptable in protein–ligand interaction (Table 1). The RMSDs of our ligands with heavy atoms are similar to crystal structure resolution which is higher than 1.65 Å and is accurately ordered and exactly fitted in the electron density map. Therefore, RMSDs obtained from MD simulation also showed the structure stability during simulation (each ligand has remained constant and has a constant range of RMSDs). From these observations, we assume that our ligand RMSDs (0.25–1 nm) showed similar stability as crystal ligand pose has in resolutions.
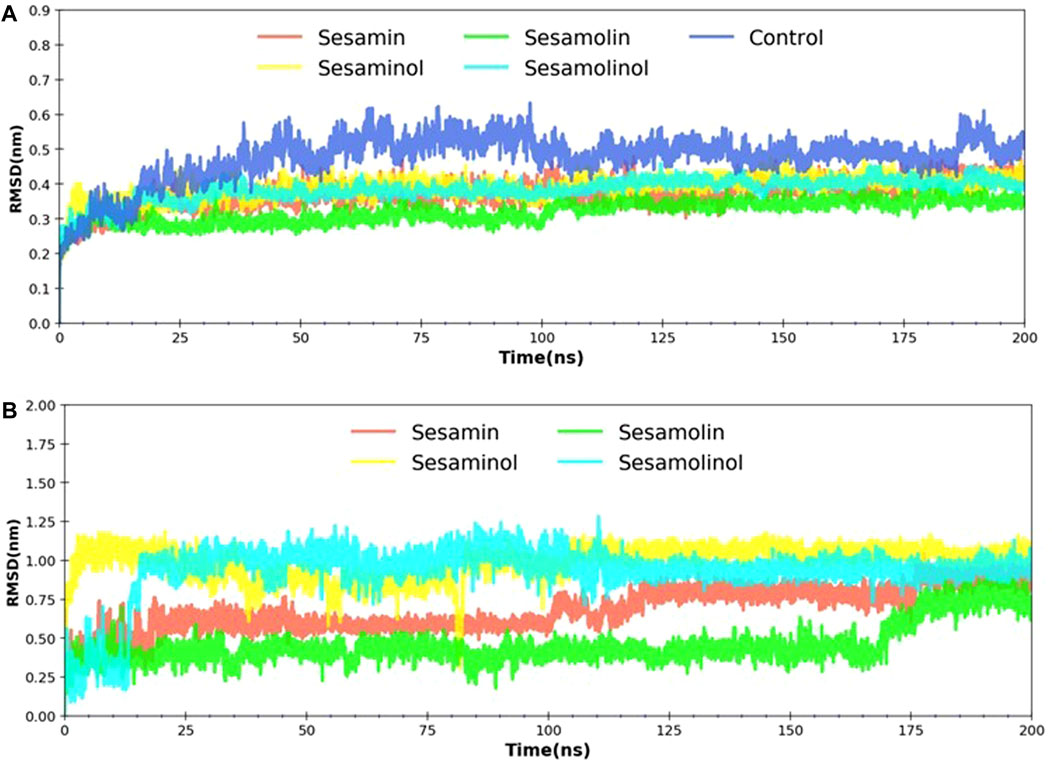
FIGURE 4. RMSD analysis. (A) Backbone RMSD plot of docking complexes; and (B) ligand RMSD plot of complexes [Mpro – sesamin complex (red), Mpro – sesamolin complex (green), Mpro – sesaminol complex (yellow), Mpro – sesamolinol complex (cyan), and control (blue)].
Root-Mean-Square Fluctuation
In order to determine the individual residue flexibility of the system with the time, RMSF was calculated, in which high fluctuation score indicates more flexibility and unstable bonds, while a low score reflects well-structured regions in the protein–ligand complexes (Gajula et al., 2016). The RMSF of alpha-carbon atoms of all system was investigated and is given in Figure 5. All the five systems (control, Mpro–sesamin, Mpro–sesaminol, Mpro–sesamolin, and Mpro–sesamolinol complexes) demonstrated almost a similar pattern of fluctuation across the whole structure during simulation. The average RMSF values of control, Mpro– sesamin, Mpro– sesaminol, Mpro– sesamolin, and Mpro– sesamolinol complexes were ∼0.25, ∼0.20, ∼0.23, ∼0.21, and ∼0.35 nm, respectively. These values revealed that all the subjected docking complexes exhibit relatively less conformation fluctuation than the control system. These less fluctuations of the docking complexes suggested that the residues distributed across the active site of Mpro interact with sesamin, sesaminol, sesamolin, and sesamolinol in a significant manner.
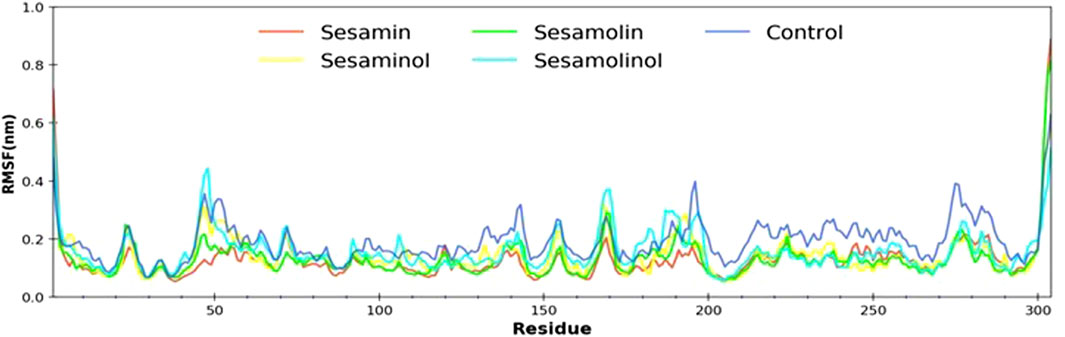
FIGURE 5. Calculated RMSF plot of docking complexes[Mpro – sesamin complex (red), Mpro – sesamolin complex (green), Mpro – sesaminol complex (yellow), Mpro – sesamolinol complex (cyan), and control (blue)].
Hydrogen Bond Analysis
Hydrogen bonds play an essential role in establishing molecular interactions of biological systems. The molecular interaction between Mpro and sesame-derived bioactive molecules was explored by the secondary structure changes, which is, in turn, regulated by a number of hydrogen bonds. For selected complexes (Mpro– sesamin, Mpro– sesaminol, Mpro– sesamolin, and Mpro– sesamolinol), a number of formed hydrogen bonds were calculated throughout the MD simulation on the scale of 200 ns. The number of hydrogen bonds and hydrogen bond distribution is represented in Figure 6. In complex with sesaminol (yellow) and sesamolinol (cyan), the numbers of hydrogen bonds were three, with few conformations showing up to 4 hydrogen bonds throughout the simulations. Sesamin (red) and sesamolin (green) have a constant range of hydrogen bonds between two and three in whole simulation. These results showed that the screened natural metabolites were able to maintain a strong interaction with a pocket site and suggested that all four docking complexes were stable throughout the simulation.
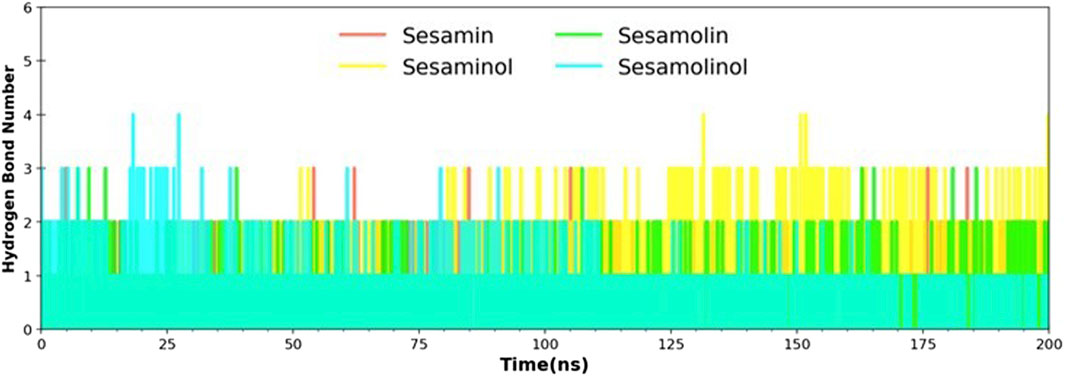
FIGURE 6. Hydrogen bond analysis of docking complexes [Mpro – sesamin complex (red), Mpro – sesamolin complex (green), Mpro – sesaminol complex (yellow), and Mpro – sesamolinol complex (cyan)].
Radius of Gyration, and Solvent Accessible Surface Area Analysis
MD trajectories corresponding to four complexes (Mpro– sesamin, Mpro– sesaminol, Mpro– sesamolin, and Mpro– sesamolinol) were further investigated with the aid of Rg and SASA analysis. Rg was calculated with a primary goal to determine the compactness of the system with the time. As depicted in Figure 7A, the Rg values of all four systems with control were reported as 2.08–2.15 nm throughout the simulation. Rg value analysis affirms the stability of each system and suggested that the binding of screened natural phytochemicals does not induce structural changes during whole simulation. During simulation, SASA values were calculated to measure the receptor exposed to the solvents. It is well-documented that a higher SASA value reflects the expansion of protein volume during MD simulation (Kumar et al., 2020). Always, a low fluctuation is expected during whole simulation. Interaction with ligand compounds may influence SASA and sometimes affect the protein structure in a significant manner. The calculated SASA values showed between 130 and 148 nm2, reflecting that the binding of sesamin, sesaminol, sesamolin, and sesamolinol does not affect the folding of protein (Figure 7B). The calculated SASA values for these ligand compounds are the consent of previous reports (Kumar et al., 2020; Mishra et al., 2021) and suggested that all of the four complexes were stable after the binding of sesamin, sesaminol, sesamolin, and sesamolinol to the Mpro active site.
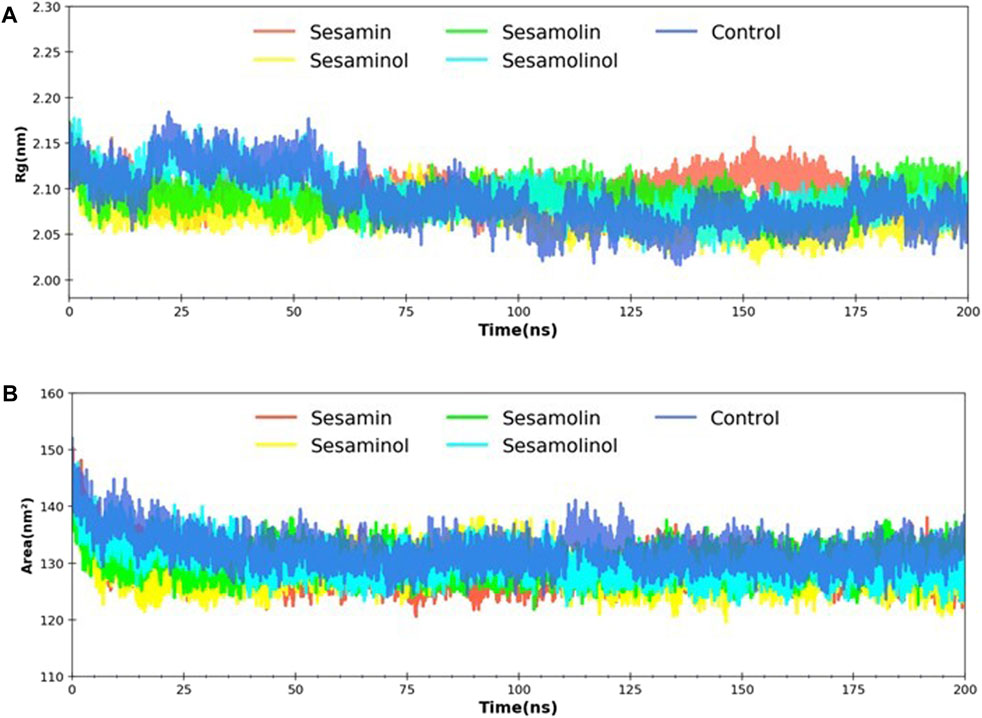
FIGURE 7. Rg and SASA analysis. (A) Predicted Rg plot of docking complexes; and (B) SASA plot of selected complexes [Mpro – sesamin complex (red), Mpro – sesamolin complex (green), Mpro – sesaminol complex (yellow), Mpro – sesamolinol complex (cyan), and control (blue)].
Estimation of Binding Free Energy
The average free binding energy of selected complexes (Mpro– sesamin, Mpro– sesaminol, Mpro– sesamolin, and Mpro– sesamolinol) was calculated by using a python script MmPbSaStat.py embedded in g_mmpbsa package. The molecular mechanic/Poisson–Boltzmann surface area (MM/PBSA) is one of the popular and accurate methods to estimate the ligand binding affinities in the protein system. To calculate the binding free energy, we have utilized the steps previously described (Gajula et al., 2016; Jee et al., 2017). The MM/PBSA-based binding energy score extracted after the systematical calculation is provided in Table 3. The cumulative sum of different energies such as van der Walls, electrostatic, polar solvation, and SASA is presented as the final binding energy. All types of the energy significantly contributed to the molecular interaction between the ligand compounds and Mpro. The evaluated binding free energy of screened molecules exhibited as sesamin (−145.511 ± 17.054 kJ/mol), sesamolin (−211.240 ± 14.034 kJ/mol), sesaminol (−149.078 ± 9.043 kJ/mol) and sesamolinol (−199.110 ± 15.881 kJ/mol). The negative values of the binding energy reflect that the targeted compound favorably interact with the receptor protein. As compared with other screened compounds, the sesamolin (−211.240 ± 14.034 kJ/mol) showed the maximum negative binding energy. The MM/PBSA results clearly suggest that sesamolinol (−199.110 ± 15.881 kJ/mol) possessed the second least binding energy. These natural compounds with the maximum negative binding energy and better binding affinity could be utilized as potential inhibitors against the Mpro of COVID-19.

TABLE 3. Calculated total binding energy, van der Waals energy, electrostatic energy, polar solvation energy, and SASA energy of the docking complexes.
Conclusion
The inhibition of Mpro protein represents a promising strategy for controlling viral replication leading to discovery of potential drug candidates. The current extensive study concludes four phytochemicals, namely, sesamin, sesaminol, sesamolin, and sesamolinol as potential inhibitors against the Mpro of SARS-CoV-2. The integrated molecular docking and MD simulation study revealed that these bioactive molecules form a very stable complex with Mpro that shows excellent binding affinities higher than other sesame-derived molecules. Docking complexes of these natural metabolites with Mpro showed a stable conformation on 200 ns, which is further supported by the results of binding free energy. Moreover, the proposed potential inhibitors also meet the criteria of drug likeness based on Lipinski’s rule of five and ADME properties. The inhibitory effect of these sesame-derived natural compounds against the Mpro of SARS-CoV-2 may also be further validated using a plethora of in vitro and in vivo experiments. The current study suggested that the screened phytochemicals (sesamin, sesaminol, sesamolin, and sesamolinol) have shown enough potential to inhibit the Mpro and may be utilized as effective drug candidates for the development of new treatment against COVID-19 infection.
Data Availability Statement
The datasets presented in this study can be found in online repositories. The names of the repository/repositories and accession number(s) can be found in the article/Supplementary Material.
Author Contributions
AK and UA contributed to conception, design, and data acquisition. AK drafted the manuscript. DM contributed to data analysis. UA, RY, AR, and DK proofread the final manuscript. All authors gave final approval and agreed to be accountable for all aspects of the work.
Funding
This research was funded by the Department of Biotechnology, Ministry of Science and Technology, government of India (grant number BT/Ag/Network/Sesame/2019-20).
Conflict of Interest
The authors declare that the research was conducted in the absence of any commercial or financial relationships that could be construed as a potential conflict of interest.
Publisher’s Note
All claims expressed in this article are solely those of the authors and do not necessarily represent those of their affiliated organizations, or those of the publisher, the editors, and the reviewers. Any product that may be evaluated in this article, or claim that may be made by its manufacturer, is not guaranteed or endorsed by the publisher.
Acknowledgments
The authors are thankful to Gourav Choudhir, Indian Institute of Technology, Delhi, for his technical support in molecular dynamics simulations. The authors are also thankful to Indian Council of Agricultural Research, Ministry of Agriculture and Farmers’ Welfare, government of India, for use of Advanced Super Computing Hub for Omics Knowledge in Agriculture (ASHOKA) facility.
Supplementary Material
The Supplementary Material for this article can be found online at: https://www.frontiersin.org/articles/10.3389/fchem.2021.744376/full#supplementary-material
References
Aanouz, I., Belhassan, A., El-Khatabi, K., Lakhlifi, T., El-ldrissi, M., and Bouachrine, M. (2020). Moroccan Medicinal Plants as Inhibitors against SARS-CoV-2 Main Protease: Computational Investigations. J. Biomol. Struct. Dyn. 39, 2971–2979. doi:10.1080/07391102.2020.1758790
Abdelli, I., Hassani, F., Bekkel Brikci, S., and Ghalem, S. (2021). In Silico study the Inhibition of Angiotensin Converting Enzyme 2 Receptor of COVID-19 by Ammoides Verticillata Components Harvested from Western Algeria. J. Biomol. Struct. Dyn. 39, 1–14. doi:10.1080/07391102.2020.1763199
Abraham, M. J., and Gready, J. E. (2011). Optimization of Parameters for Molecular Dynamics Simulation Using Smooth Particle-Mesh Ewald in GROMACS 4.5. J. Comput. Chem. 32, 2031–2040. doi:10.1002/jcc.21773
Abraham, M. J., Murtola, T., Schulz, R., Páll, S., Smith, J. C., Hess, B., et al. (2015). GROMACS: High Performance Molecular Simulations through Multi-Level Parallelism from Laptops to Supercomputers. SoftwareX 1–2, 19–25. doi:10.1016/j.softx.2015.06.001
Ahuja, A., Tyagi, P. K., Tyagi, S., Kumar, A., Kumar, M., and Sharifi-Rad, J. (2021). Potential of Pueraria Tuberosa (Willd.) DC. To rescue Cognitive Decline Associated with BACE1 Protein of Alzheimer's Disease on Drosophila Model: An Integrated Molecular Modeling and In Vivo Approach. Int. J. Biol. Macromolecules 179, 586–600. doi:10.1016/j.ijbiomac.2021.03.032
Aldeghi, M., Bodkin, M. J., Knapp, S., and Biggin, P. C. (2017). Statistical Analysis on the Performance of Molecular Mechanics Poisson-Boltzmann Surface Area versus Absolute Binding Free Energy Calculations: Bromodomains as a Case Study. J. Chem. Inf. Model. 57, 2203–2221. doi:10.1021/acs.jcim.7b00347
Allam, A. E., Amen, Y., Ashour, A., Assaf, H. K., Hassan, H. A., Abdel-Rahman, I. M., et al. (2021). In Silico study of Natural Compounds from Sesame against COVID-19 by Targeting Mpro, PLpro and RdRp. RSC Adv. 11, 22398–22408. doi:10.1039/D1RA03937G
Arden, K. E., Nissen, M. D., Sloots, T. P., and Mackay, I. M. (2005). New Human Coronavirus, HCoV-NL63, Associated with Severe Lower Respiratory Tract Disease in Australia. J. Med. Virol. 75, 455–462. doi:10.1002/jmv.20288
Backer, J. A., Klinkenberg, D., and Wallinga, J. (2020). Incubation Period of 2019 Novel Coronavirus (2019-nCoV) Infections Among Travellers from Wuhan, China, 20-28 January 2020. Eurosurveillance 25, 2000062. doi:10.2807/1560-7917.ES.2020.25.5.2000062
Bello, M., Martínez-Muñoz, A., and Balbuena-Rebolledo, I. (2020). Identification of Saquinavir as a Potent Inhibitor of Dimeric SARS-CoV2 Main Protease through MM/GBSA. J. Mol. Model. 26, 340. doi:10.1007/s00894-020-04600-4
Berman, H. M. (2000). The Protein Data Bank. Nucleic Acids Res. 28, 235–242. doi:10.1093/nar/28.1.235
Bhat, K. V., Kumari, R., Pathak, N., and Rai, A. K. (2014). Value Addition in Sesame: A Perspective on Bioactive Components for Enhancing Utility and Profitability. Phcog Rev. 8, 147. doi:10.4103/0973-7847.134249
Burley, S. K., Berman, H. M., Bhikadiya, C., Bi, C., Chen, L., Di Costanzo, L., et al. (2018). RCSB Protein Data Bank: Biological Macromolecular Structures Enabling Research and Education in Fundamental Biology, Biomedicine, Biotechnology and Energy. Nucleic Acids Res. 47, D464–D474. doi:10.1093/nar/gky1004
Chang, C.-k., Lo, S.-C., Wang, Y.-S., and Hou, M.-H. (2016). Recent Insights into the Development of Therapeutics against Coronavirus Diseases by Targeting N Protein. Drug Discov. Today 21, 562–572. doi:10.1016/j.drudis.2015.11.015
Chang, Y.-C., Tung, Y.-A., Lee, K.-H., Chen, T.-F., Hsiao, Y.-C., Chang, H.-C., et al. (2020). Potential Therapeutic Agents for COVID-19 Based on the Analysis of Protease and RNA Polymerase Docking. Preprints, 2020020242. doi:10.20944/preprints202002.0242.v1
Chen, T., Wu, D., Chen, H., Yan, W., Yang, D., Chen, G., et al. (2020a). Clinical Characteristics of 113 Deceased Patients with Coronavirus Disease 2019: Retrospective Study. BMJ 368, m1091. doi:10.1136/bmj.m1091
Chen, Y., Liu, Q., and Guo, D. (2020b). Emerging Coronaviruses: Genome Structure, Replication, and Pathogenesis. J. Med. Virol. 92, 418–423. doi:10.1002/jmv.25681
Cheng, V. C. C., Lau, S. K. P., Woo, P. C. Y., and Yuen, K. Y. (2007). Severe Acute Respiratory Syndrome Coronavirus as an Agent of Emerging and Reemerging Infection. Clin. Microbiol. Rev. 20, 660–694. doi:10.1128/cmr.00023-07
Chikhale, R. V., Gurav, S. S., Patil, R. B., Sinha, S. K., Prasad, S. K., Shakya, A., et al. (2020a). Sars-cov-2 Host Entry and Replication Inhibitors from Indian Ginseng: an In-Silico Approach. J. Biomol. Struct. Dyn. 39, 4510–4521. doi:10.1080/07391102.2020.1778539
Chikhale, R. V., Sinha, S. K., Patil, R. B., Prasad, S. K., Shakya, A., Gurav, N., et al. (2020b). In-silico Investigation of Phytochemicals from Asparagus racemosus as Plausible Antiviral Agent in COVID-19. J. Biomol. Struct. Dyn. 39, 5033–5047. doi:10.1080/07391102.2020.1784289
Chung, B.-H., Lee, J. J., Kim, J.-D., Jeoung, D., Lee, H., Choe, J., et al. (2010). Angiogenic Activity of Sesamin through the Activation of Multiple Signal Pathways. Biochem. Biophysical Res. Commun. 391, 254–260. doi:10.1016/j.bbrc.2009.11.045
Contini, A. (2020). Virtual Screening of an FDA Approved Drugs Database on Two COVID-19 Coronavirus Proteins. Life Sci. 251, 117627. doi:10.26434/chemrxiv.11847381.v1
Daina, A., Michielin, O., and Zoete, V. (2017). SwissADME: A Free Web Tool to Evaluate Pharmacokinetics, Drug-Likeness and Medicinal Chemistry Friendliness of Small Molecules. Sci. Rep. 7, 42717. doi:10.1038/srep42717
Dalibalta, S., Majdalawieh, A. F., and Manjikian, H. (2020). Health Benefits of Sesamin on Cardiovascular Disease and its Associated Risk Factors. Saudi Pharm. J. 28, 1276–1289. doi:10.1016/j.jsps.2020.08.018
Dar, A. A., and Arumugam, N. (2013). Lignans of Sesame: Purification Methods, Biological Activities and Biosynthesis - A Review. Bioorg. Chem. 50, 1–10. doi:10.1016/j.bioorg.2013.06.009
Das, S., Sarmah, S., Lyndem, S., and Singha Roy, A. (2020). An Investigation into the Identification of Potential Inhibitors of SARS-CoV-2 Main Protease Using Molecular Docking Study. J. Biomol. Struct. Dyn. 39, 3347–3357. doi:10.1080/07391102.2020.1763201
de Wit, E., van Doremalen, N., Falzarano, D., and Munster, V. J. (2016). SARS and MERS: Recent Insights into Emerging Coronaviruses. Nat. Rev. Microbiol. 14, 523–534. doi:10.1038/nrmicro.2016.81
Dravie, E. E., Kortei, N. K., Essuman, E. K., Tettey, C. O., Boakye, A. A., and Hunkpe, G. (2020). Antioxidant, Phytochemical and Physicochemical Properties of Sesame Seed (Sesamum indicum L). Scientific Afr. 8, e00349. doi:10.1016/j.sciaf.2020.e00349
Elfiky, A. A., and Azzam, E. B. (2020). Novel Guanosine Derivatives against MERS CoV Polymerase: An In Silico Perspective. J. Biomol. Struct. Dyn. 39, 2923–2931. doi:10.1080/07391102.2020.1758789
Elfiky, A. A. (2020). SARS-CoV-2 RNA Dependent RNA Polymerase (RdRp) Targeting: An In Silico Perspective. J. Biomol. Struct. Dyn. 39, 3204–3212. doi:10.1080/07391102.2020.1761882
Enayatkhani, M., Hasaniazad, M., Faezi, S., Gouklani, H., Davoodian, P., Ahmadi, N., et al. (2020). Reverse Vaccinology Approach to Design a Novel Multi-Epitope Vaccine Candidate against COVID-19: an In Silico Study. J. Biomol. Struct. Dyn. 39, 2857–2872. doi:10.1080/07391102.2020.1756411
Ertl, P., and Schuffenhauer, A. (2009). Estimation of Synthetic Accessibility Score of Drug-like Molecules Based on Molecular Complexity and Fragment Contributions. J. Cheminform 1, 8. doi:10.1186/1758-2946-1-8
Fanhchaksai, K., Kodchakorn, K., Pothacharoen, P., and Kongtawelert, P. (2015). Effect of Sesamin against Cytokine Production from Influenza Type A H1N1-Induced Peripheral Blood Mononuclear Cells: Computational and Experimental Studies In Vitro. Cell. Dev. Biol. 52, 107–119. doi:10.1007/s11626-015-9950-7
Gajula, M., Kumar, A., and Ijaq, J. (2016). Protocol for Molecular Dynamics Simulations of Proteins. Bio-protocol 85, 159–166. doi:10.21769/bioprotoc.2051
Gonzalez-Paz, L. A., Lossada, C. A., Moncayo, L. S., Romero, F., Paz, J. L., Vera-Villalobos, J., et al. (2020). Theoretical Molecular Docking Study of the Structural Disruption of the Viral 3CL-Protease of COVID19 Induced by Binding of Capsaicin, Piperine and Curcumin Part 1: A Comparative Study with Chloroquine and Hydrochloroquine Two Antimalaric Drugs. Research Square. doi:10.21203/rs.3.rs-21206/v1
Gordon, D. E., Jang, G. M., Bouhaddou, M., Xu, J., Obernier, K., O’Meara, M. J., et al. (2020). A SARS-CoV-2-Human Protein-Protein Interaction Map Reveals Drug Targets and Potential Drug-Repurposing. Nature 583, 459–468. doi:10.1101/2020.03.22.002386
Grougnet, R., Magiatis, P., Laborie, H., Lazarou, D., Papadopoulos, A., and Skaltsounis, A.-L. (2011). Sesamolinol Glucoside, Disaminyl Ether, and Other Lignans from Sesame Seeds. J. Agric. Food Chem. 60, 108–111. doi:10.1021/jf2040687
Guex, N., and Peitsch, M. C. (1997). SWISS-MODEL and the Swiss-Pdb Viewer: An Environment for Comparative Protein Modeling. Electrophoresis 18, 2714–2723. doi:10.1002/elps.1150181505
Gunda, S. K., P, H. K., Kumar, A., Kishor, P. B. K., and S, A. K. (2021). Natural Xanthone Compounds as Promising Drug Candidates against COVID-19 - an Integrated Molecular Docking and Dynamics Simulation Study. Research Square. doi:10.21203/rs.3.rs-102884/v2
Gupta, A., Madhavan, M. V., Sehgal, K., Nair, N., Mahajan, S., Sehrawat, T. S., et al. (2020). Extrapulmonary Manifestations of COVID-19. Nat. Med. 26, 1017–1032. doi:10.1038/s41591-020-0968-3
Hasan, A., Paray, B. A., Hussain, A., Qadir, F. A., Attar, F., Aziz, F. M., et al. (2020). A Review on the Cleavage Priming of the Spike Protein on Coronavirus by Angiotensin-Converting Enzyme-2 and Furin. J. Biomol. Struct. Dyn. 39, 3025–3033. doi:10.1080/07391102.2020.1754293
Helmy, Y. A., Fawzy, M., Elaswad, A., Sobieh, A., Kenney, S. P., and Shehata, A. A. (2020). The COVID-19 Pandemic: A Comprehensive Review of Taxonomy, Genetics, Epidemiology, Diagnosis, Treatment, and Control. Jcm 9, 1225. doi:10.3390/jcm9041225
Hess, B., Bekker, H., Berendsen, H. J. C., and Fraaije, J. G. E. M. (1997). LINCS: A Linear Constraint Solver for Molecular Simulations. J. Comput. Chem. 18, 1463–1472. doi:10.1002/(sici)1096-987x(199709)18:12<1463:aid-jcc4>3.0.co;2-h
Hess, B. (2007). P-LINCS: A Parallel Linear Constraint Solver for Molecular Simulation. J. Chem. Theor. Comput. 4, 116–122. doi:10.1021/ct700200b
Hulswit, R. J. G., de Haan, C. A. M., and Bosch, B.-J. (2016). Coronavirus Spike Protein and Tropism Changes. Adv. Virus. Res. 96, 29–57. doi:10.1016/bs.aivir.2016.08.004
Islam, R., Parves, M. R., Paul, A. S., Uddin, N., Rahman, M. S., Mamun, A. A., et al. (2020). A Molecular Modeling Approach to Identify Effective Antiviral Phytochemicals against the Main Protease of SARS-CoV-2. J. Biomol. Struct. Dyn. 39, 3213–3224. doi:10.1080/07391102.2020.1761883
Jamwal, S., Gautam, A., Elsworth, J., Kumar, M., Chawla, R., and Kumar, P. (2020). An Updated Insight into the Molecular Pathogenesis, Secondary Complications and Potential Therapeutics of COVID-19 Pandemic. Life Sci. 257, 118105. doi:10.1016/j.lfs.2020.118105
Jee, B., Kumar, S., Yadav, R., Singh, Y., Kumar, A., and Sharma, N. (2017). Ursolic Acid and Carvacrol May Be Potential Inhibitors of Dormancy Protein Small Heat Shock protein16.3 of Mycobacterium tuberculosis. J. Biomol. Struct. Dyn. 36, 3434–3443. doi:10.1080/07391102.2017.1389305
Jin, Z., Zhao, Y., Sun, Y., Zhang, B., Wang, H., Wu, Y., et al. (2020). Structural Basis for the Inhibition of SARS-CoV-2 Main Protease by Antineoplastic Drug Carmofur. Nat. Struct. Mol. Biol. 27, 529–532. doi:10.1038/s41594-020-0440-6
Joshi, R. S., Jagdale, S. S., Bansode, S. B., Shankar, S. S., Tellis, M. B., Pandya, V. K., et al. (2020). Discovery of Potential Multi-Target-Directed Ligands by Targeting Host-specific SARS-CoV-2 Structurally Conserved Main Protease. J. Biomol. Struct. Dyn. 39, 3099–3114. doi:10.1080/07391102.2020.1760137
Kaji, H., Matsui-Yuasa, I., Matsumoto, K., Omura, A., Kiyomoto, K., and Kojima-Yuasa, A. (2020). Sesaminol Prevents Parkinson's Disease by Activating the Nrf2-ARE Signaling Pathway. Heliyon 6, e05342. doi:10.1016/j.heliyon.2020.e05342
Kappo, A., Oyinloye, B., Ajiboye, B., Ojo, O., and Nwozo, S. (2016). Cardioprotective and Antioxidant Influence of Aqueous Extracts from Sesamum indicum Seeds on Oxidative Stress Induced by Cadmium in Wistar Rats. Phcog Mag. 12, 170. doi:10.4103/0973-1296.182155
Khan, S. A., Zia, K., Ashraf, S., Uddin, R., and Ul-Haq, Z. (2020). Identification of Chymotrypsin-like Protease Inhibitors of SARS-CoV-2 via Integrated Computational Approach. J. Biomol. Struct. Dyn. 39, 2607–2616. doi:10.1080/07391102.2020.1751298
Kim, S., Chen, J., Cheng, T., Gindulyte, A., He, J., He, S., et al. (2020). PubChem in 2021: New Data Content and Improved Web Interfaces. Nucleic Acids Res. 49, D1388–D1395. doi:10.1093/nar/gkaa971
Kodchakorn, K., Poovorawan, Y., Suwannakarn, K., and Kongtawelert, P. (2020). Molecular Modelling Investigation for Drugs and Nutraceuticals Against Protease of SARS-CoV-2. J. Mol. Graphics Model. 101, 107717. doi:10.1016/j.jmgm.2020.107717
Krupanidhi, S., Abraham Peele, K., Venkateswarulu, T. C., Ayyagari, V. S., Nazneen Bobby, M., John Babu, D., et al. (2020). Screening of Phytochemical Compounds of Tinospora Cordifolia for Their Inhibitory Activity on SARS-CoV-2: an In Silico Study. J. Biomol. Struct. Dyn. 39, 5799–5803. doi:10.1080/07391102.2020.1787226
Kumar, A., Choudhir, G., Shukla, S. K., Sharma, M., Tyagi, P., Bhushan, A., et al. (2020). Identification of Phytochemical Inhibitors against Main Protease of COVID-19 Using Molecular Modeling Approaches. J. Biomol. Struct. Dyn. 39, 3760–3770. doi:10.1080/07391102.2020.1772112
Kumar, A., Kumar, R., Sharma, M., Kumar, U., Gajula, M., and Singh, K. (2018). Uttarakhand Medicinal Plants Database (UMPDB): A Platform for Exploring Genomic, Chemical, and Traditional Knowledge. Data 3, 7. doi:10.3390/data3010007
Kumari, R., Kumar, R., and Lynn, A. (2014). g_mmpbsa-A GROMACS Tool for High-Throughput MM-PBSA Calculations. J. Chem. Inf. Model. 54, 1951–1962. doi:10.1021/ci500020m
Kutzner, C., Páll, S., Fechner, M., Esztermann, A., Groot, B. L., and Grubmüller, H. (2019). More Bang for Your Buck: Improved Use of GPU Nodes for GROMACS 2018. J. Comput. Chem. 40, 2418–2431. doi:10.1002/jcc.26011
Lee, N., Allen Chan, K. C., Hui, D. S., Ng, E. K. O., Wu, A., Chiu, R. W. K., et al. (2004). Effects of Early Corticosteroid Treatment on Plasma SARS-Associated Coronavirus RNA Concentrations in Adult Patients. J. Clin. Virol. 31, 304–309. doi:10.1016/j.jcv.2004.07.006
Lipinski, C. A., Lombardo, F., Dominy, B. M., and Feeney, P. J. (2001). Experimental and computational approaches to estimate solubility and permeability in drug discovery and development settings. Adv. Drug Deliv. Rev. 46, 3–26. doi:10.1016/s0169-409x(00)00129-0
Lipinski, C. A. (2004). Lead- and Drug-like Compounds: the Rule-Of-Five Revolution. Drug Discov. Today Tech. 1, 337–341. doi:10.1016/j.ddtec.2004.11.007
Liu, X.-h., Zhang, X., Lu, Z.-h., Zhu, Y.-s., and Wang, T. (2021). Potential Molecular Targets of Nonstructural Proteins for the Development of Antiviral Drugs against SARS-CoV-2 Infection. Biomed. Pharmacother. 133, 111035. doi:10.1016/j.biopha.2020.111035
Mackenzie, J. S., and Smith, D. W. (2020). COVID-19: A Novel Zoonotic Disease Caused by a Coronavirus from China: what We Know and what We Don’t. Microbiol. Aust. 41, 45. doi:10.1071/ma20013
Mahendra Kumar, C., and Singh, S. A. (2014). Bioactive Lignans from Sesame (Sesamum indicum L.): Evaluation of Their Antioxidant and Antibacterial Effects for Food Applications. J. Food Sci. Technol. 52, 2934–2941. doi:10.1007/s13197-014-1334-6
Majdalawieh, A. F., Massri, M., and Nasrallah, G. K. (2017). A Comprehensive Review on the AnticancerProperties and Mechanisms of Action of Sesamin, a Lignan in Sesame Seeds (Sesamum indicum). Eur. J. Pharmacol. 815, 512–521. doi:10.1016/j.ejphar.2017.10.020
Matveeva, T., Khafizova, G., and Sokornova, S. (2020). In Search of Herbal Anti-SARS-cov2 Compounds. Front. Plant Sci. 11, 589998. doi:10.3389/fpls.2020.589998
Maurya, D. K., and Sharma, D. (2020). Evaluation of Traditional Ayurvedic Kadha for Prevention and Management of the Novel Coronavirus (SARS-CoV-2) Using In Silico Approach. J. Biomol. Struct. Dyn. 30, 1–16. doi:10.1080/07391102.2020.1852119
Michailidis, D., Angelis, A., Aligiannis, N., Mitakou, S., and Skaltsounis, L. (2019). Recovery of Sesamin, Sesamolin, and Minor Lignans from Sesame Oil Using Solid Support-free Liquid-Liquid Extraction and Chromatography Techniques and Evaluation of Their Enzymatic Inhibition Properties. Front. Pharmacol. 10, 723. doi:10.3389/fphar.2019.00723
Mishra, A., Pathak, Y., Kumar, A., Mishra, S. K., and Tripathi, V. (2021). Natural Compounds as Potential Inhibitors of SARS-CoV-2 Main Protease: An In-Silico Study. Asian Pac. J. Trop. Biomed. 11, 155–163. doi:10.4103/2221-1691.310202
Mitra, D., Verma, D., Mahakur, B., Kamboj, A., Srivastava, R., Gupta, S., et al. (2021). Molecular Docking and Simulation Studies of Natural Compounds of Vitex negundo L. Against Papain-Like Protease (PLpro) of SARS CoV-2 (Coronavirus) to Conquer the Pandemic Situation in the World. J. Biomol. Struct. Dyn. 18, 1–22. doi:10.1080/07391102.2021.1873185
Miyahara, Y., Hibasami, H., Katsuzaki, H., Imai, K., Osawa, T., Ina, K., et al. (2001). Sesaminol from Sesame Seed Induces Apoptosis in Human Lymphoid Leukemia Molt 4B Cells. Int. J. Mol. Med. 7, 485–488. doi:10.3892/ijmm.7.5.485
Morris, G. M., Huey, R., Lindstrom, W., Sanner, M. F., Belew, R. K., Goodsell, D. S., et al. (2009). AutoDock4 and AutoDockTools4: Automated Docking with Selective Receptor Flexibility. J. Comput. Chem. 30, 2785–2791. doi:10.1002/jcc.21256
Muhammad, I. A., Muangchoo, K., Muhammad, A., Ajingi, Y. S., Muhammad, I. Y., Umar, I. D., et al. (2020). A Computational Study to Identify Potential Inhibitors of SARS-CoV-2 Main Protease (Mpro) from Eucalyptus Active Compounds. Computation 8, 79. doi:10.3390/computation8030079
Nagendra Prasad, M. N., Sanjay, K. R., and Prasad, D. (2012). A Review on Nutritional and Nutraceutical Properties of Sesame. J. Nutr. Food Sci. 02, 2. doi:10.4172/2155-9600.1000127
O’Boyle, N. M., Banck, M., James, C. A., Morley, C., Vandermeersch, T., and Hutchison, G. R. (2011). Open Babel: An Open Chemical Toolbox. J. Cheminform 3, 33. doi:10.1186/1758-2946-3-33
Ou, T., Mou, H., Zhang, L., Ojha, A., Choe, H., and Farzan, M. (2021). Hydroxychloroquine-mediated Inhibition of SARS-CoV-2 Entry is Attenuated by TMPRSS2. PLOS Pathog. 17, e1009212. doi:10.1371/journal.ppat.1009212
Pandey, A. K., and Verma, S. (2020). An In-Silico Evaluation of Dietary Components for Structural Inhibition of SARS-Cov-2 Main Protease. J. Biomol. Struct. Dyn. 0, 1–7. doi:10.1080/07391102.2020.1809522
Pant, S., Singh, M., Ravichandiran, V., Murty, U. S. N., and Srivastava, H. K. (2020). Peptide-like and Small-Molecule Inhibitors against Covid-19. J. Biomol. Struct. Dyn. 39, 2904–2913. doi:10.1080/07391102.2020.1757510
Park, J.-Y., Ko, J.-A., Kim, D. W., Kim, Y. M., Kwon, H.-J., Jeong, H. J., et al. (2015). Chalcones Isolated fromAngelica Keiskeiinhibit Cysteine Proteases of SARS-CoV. J. Enzyme Inhib. Med. Chem. 31, 23–30. doi:10.3109/14756366.2014.1003215
Park, S.-H., Ryu, S.-N., Bu, Y., Kim, H., Simon, J. E., and Kim, K.-S. (2010). Antioxidant Components as Potential Neuroprotective Agents in Sesame (Sesamum indicumL.). Food Rev. Int. 26, 103–121. doi:10.1080/87559120903564464
Pathak, N., Bhaduri, A., and Rai, A. K. (2019). “Sesame: Bioactive Compounds and Health Benefits,” in Bioactive Molecules in Food (Switzerland: Springer International Publishing), 181–200. doi:10.1007/978-3-319-78030-6_59
Pathak, Y., Mishra, A., Choudhir, G., Kumar, A., and Tripathi, V. (2021). Rifampicin and Letermovir as Potential Repurposed Drug Candidate for COVID-19 Treatment: Insights from an In-Silico Study. Pharmacol. Rep. 73, 926–938. doi:10.1007/s43440-021-00228-0
Prasanth, D. S. N. B. K., Murahari, M., Chandramohan, V., Bhavya, G., Lakshmana Rao, A., Panda, S. P., et al. (2021). In-silico Strategies of Some Selected Phytoconstituents from Melissa Officinalis as SARS CoV-2 Main Protease and Spike Protein (COVID-19) Inhibitors. Mol. Simulation 47, 457–470. doi:10.1080/08927022.2021.1880576
Raj, V., Park, J. G., Cho, K.-H., Choi, P., Kim, T., Ham, J., et al. (2021). Assessment of Antiviral Potencies of Cannabinoids against SARS-CoV-2 Using Computational and In Vitro Approaches. Int. J. Biol. Macromolecules 168, 474–485. doi:10.1016/j.ijbiomac.2020.12.020
Romeo, I., Mesiti, F., Lupia, A., and Alcaro, S. (2021). Current Updates on Naturally Occurring Compounds Recognizing SARS-CoV-2 Druggable Targets. Molecules 26, 632. doi:10.3390/molecules26030632
Rothe, C., Schunk, M., Sothmann, P., Bretzel, G., Froeschl, G., Wallrauch, C., et al. (2020). Transmission of 2019-nCoV Infection from an Asymptomatic Contact in Germany. N. Engl. J. Med. 382, 970–971. doi:10.1056/nejmc2001468
Rout, J., Swain, B. C., and Tripathy, U. (2020). In Silico investigation of Spice Molecules as Potent Inhibitor of SARS-CoV-2. J. Biomol. Struct. Dyn. 17, 1–15. doi:10.1080/07391102.2020.1819879
Russell, T. W., Golding, N., Golding, N., Hellewell, J., Abbott, S., Wright, L., et al. (2020). Reconstructing the Early Global Dynamics of Under-ascertained COVID-19 Cases and Infections. BMC Med. 18, 332. doi:10.1186/s12916-020-01790-9
Sargsyan, K., Grauffel, C., and Lim, C. (2017). How Molecular Size Impacts RMSD Applications in Molecular Dynamics Simulations. J. Chem. Theor. Comput. 13, 1518–1524. doi:10.1021/acs.jctc.7b00028
Schüttelkopf, A. W., and van Aalten, D. M. F. (2004). PRODRG: a Tool for High-Throughput Crystallography of Protein-Ligand Complexes. Acta Crystallogr. D Biol. Cryst. 60, 1355–1363. doi:10.1107/s0907444904011679
Shamsi, A., Mohammad, T., Anwar, S., Amani, S., Khan, M. S., Husain, F. M., et al. (2021). Potential Drug Targets of SARS-CoV-2: From Genomics to Therapeutics. Int. J. Biol. Macromolecules 177, 1–9. doi:10.1016/j.ijbiomac.2021.02.071
Shi, Y., Yu, X., Zhao, H., Wang, H., Zhao, R., and Sheng, J. (2020). Host Susceptibility to Severe COVID-19 and Establishment of a Host Risk Score: Findings of 487 Cases Outside Wuhan. Crit. Care 24, 108. doi:10.1186/s13054-020-2833-7
Singh, G., Tiwari, A., Choudhir, G., P, H., Kumar, A., and Sharma, S. (2021). Unraveling the Potential Role of Bioactive Molecules Produced by Trichoderma spp. As Inhibitors of Tomatinase Enzyme Having an Important Role in Wilting Disease: An In-Silico Approach. J. Biomol. Struct. Dyn. 15, 1–10. doi:10.1080/07391102.2021.1898476
Sinha, S. K., Shakya, A., Prasad, S. K., Singh, S., Gurav, N. S., Prasad, R. S., et al. (2020). An In-Silico Evaluation of Different Saikosaponins for Their Potency Against SARS-CoV-2 Using NSP15 and Fusion Spike Glycoprotein as Targets. J. Biomol. Struct. Dyn. 39, 3244–3255. doi:10.1080/07391102.2020.1762741
Su, S., Wong, G., Shi, W., Liu, J., Lai, A. C. K., Zhou, J., et al. (2016). Epidemiology, Genetic Recombination, and Pathogenesis of Coronaviruses. Trends Microbiol. 24, 490–502. doi:10.1016/j.tim.2016.03.003
Sultana, J., Crisafulli, S., Gabbay, F., Lynn, E., Shakir, S., and Trifirò, G. (2020). Challenges for Drug Repurposing in the COVID-19 Pandemic Era. Front. Pharmacol. 11, 588654. doi:10.3389/fphar.2020.588654
Suravajhala, R., Parashar, A., Malik, B., Nagaraj, A. V., Padmanaban, G., Kavi Kishor, P., et al. (2020). Comparative Docking Studies on Curcumin with COVID-19 Proteins. Netw. Model. Anal. Health Inform. Bioinform 10, 44. doi:10.20944/preprints202005.0439.v1
Tang, W., Hu, T., Hu, B., Jin, C., Wang, G., Xie, C., et al. (2020). Prevalence and Correlates of PTSD and Depressive Symptoms One Month after the Outbreak of the COVID-19 Epidemic in a Sample of home-quarantined Chinese university Students. J. Affective Disord. 274, 1–7. doi:10.1016/j.jad.2020.05.009
Tock, M. J., Chen, W., Combrinck, S., Sandasi, M., Kamatou, G. P. P., and Viljoen, A. M. (2021). Exploring the Phytochemical Variation of Non-volatile Metabolites within Three South African Salvia Species Using UPLC-MS Fingerprinting and Chemometric Analysis. Fitoterapia 152, 104940. doi:10.1016/j.fitote.2021.104940
Tripathi, M. K., Singh, P., Sharma, S., Singh, T. P., Ethayathulla, A. S., and Kaur, P. (2020). Identification of Bioactive Molecule from Withania Somnifera (Ashwagandha) as SARS-CoV-2 Main Protease Inhibitor. J. Biomol. Struct. Dyn. 39, 5668–5681. doi:10.1080/07391102.2020.1790425
Ullrich, S., and Nitsche, C. (2020). The SARS-CoV-2 Main Protease as Drug Target. Bioorg. Med. Chem. Lett. 30, 127377. doi:10.1016/j.bmcl.2020.127377
Uncu, A. Ö., Gultekin, V., Allmer, J., Frary, A., and Doganlar, S. (2015). Genomic Simple Sequence Repeat Markers Reveal Patterns of Genetic Relatedness and Diversity in Sesame. Plant Genome 8, 0087. doi:10.3835/plantgenome2014.11.0087
Varadharajan, V., Arumugam, G. S., and Shanmugam, S. (2021). Isatin-based Virtual High Throughput Screening, Molecular Docking, DFT, QM/MM, MD and MM-PBSA Study of Novel Inhibitors of SARS-CoV-2 Main Protease. J. Biomol. Struct. Dyn. 25, 1–16. doi:10.1080/07391102.2021.1904003
Veber, D. F., Johnson, S. R., Cheng, H.-Y., Smith, B. R., Ward, K. W., and Kopple, K. D. (2002). Molecular Properties that Influence the Oral Bioavailability of Drug Candidates. J. Med. Chem. 45, 2615–2623. doi:10.1021/jm020017n
Verdoni, L., Mazza, A., Gervasoni, A., Martelli, L., Ruggeri, M., Ciuffreda, M., et al. (2020). An Outbreak of Severe Kawasaki-like Disease at the Italian Epicentre of the SARS-CoV-2 Epidemic: An Observational Cohort Study. The Lancet 395, 1771–1778. doi:10.1016/s0140-6736(20)31103-x
Wadood, A., Ahmed, N., Shah, L., Ahmad, A., Hassan, H., and Shams, S. (2013). In-silico Drug Design: An Approach Which Revolutionarised the Drug Discovery Process. OA Drug Des. Deliv. 1, 3. doi:10.13172/2054-4057-1-1-1119
Wahedi, H. M., Ahmad, S., and Abbasi, S. W. (2020). Stilbene-based Natural Compounds as Promising Drug Candidates against COVID-19. J. Biomol. Struct. Dyn. 39, 3225–3234. doi:10.1080/07391102.2020.1762743
Wang, L., Wang, Y., Ye, D., and Liu, Q. (2020a). Review of the 2019 Novel Coronavirus (SARS-CoV-2) Based on Current Evidence. Int. J. Antimicrob. Agents 55, 105948. doi:10.1016/j.ijantimicag.2020.105948
Wang, W., Xu, Y., Gao, R., Lu, R., Han, K., Wu, G., et al. (2020b). Detection of SARS-CoV-2 in Different Types of Clinical Specimens. JAMA 323, 1843–1844. doi:10.1001/jama.2020.3786
Watanabe, M., Iizumi, Y., Sukeno, M., Iizuka-Ohashi, M., Sowa, Y., and Sakai, T. (2017). The Pleiotropic Regulation of Cyclin D1 by Newly Identified Sesaminol-Binding Protein ANT2. Oncogenesis 6, e311. doi:10.1038/oncsis.2017.10
Woo, P. C. Y., Lau, S. K. P., Chu, C.-m., Chan, K.-h., Tsoi, H.-w., Huang, Y., et al. (2005). Characterization and Complete Genome Sequence of a Novel Coronavirus, Coronavirus HKU1, from Patients with Pneumonia. J. Virol. 79, 884–895. doi:10.1128/jvi.79.2.884-895.2005
Wu, A., Peng, Y., Huang, B., Ding, X., Wang, X., Niu, P., et al. (2020a). Genome Composition and Divergence of the Novel Coronavirus (2019-nCoV) Originating in China. Cell Host Microbe 27, 325–328. doi:10.1016/j.chom.2020.02.001
Wu, Y.-C., Chen, C.-S., and Chan, Y.-J. (2020b). The Outbreak of COVID-19: An Overview. J. Chin. Med. Assoc. 83, 217–220. doi:10.1097/jcma.0000000000000270
Wu, Z., and McGoogan, J. M. (2020). Characteristics of and Important Lessons from the Coronavirus Disease 2019 (COVID-19) Outbreak in China. JAMA 323, 1239. doi:10.1001/jama.2020.2648
Xu, X.-W., Wu, X.-X., Jiang, X.-G., Xu, K.-J., Ying, L.-J., Ma, C.-L., et al. (2020). Clinical Findings in a Group of Patients Infected with the 2019 Novel Coronavirus (SARS-Cov-2) outside of Wuhan, China: Retrospective Case Series. BMJ 368, m606. doi:10.1136/bmj.m606
Yu, S., and Yu, M. (2020). Severe Acute Respiratory Syndrome Coronavirus 2-induced Neurological Complications. Front. Cell Dev. Biol. 8, 8. doi:10.3389/fcell.2020.605972
Yu, W., and MacKerell, A. D. (2016). “Computer-Aided Drug Design Methods,” in Methods in Molecular Biology. (New York: Springer), 85–106. doi:10.1007/978-1-4939-6634-9_5
Yu, Y., Xu, D., Fu, S., Zhang, J., Yang, X., Xu, L., et al. (2020). Patients with COVID-19 in 19 ICUs in Wuhan, China: a Cross-Sectional Study. Crit. Care 24, 219. doi:10.1186/s13054-020-02939-x
Yuan, M., Wu, N. C., Zhu, X., Lee, C.-C. D. D., So, R. T. Y., Lv, H., et al. (2020). A Highly Conserved Cryptic Epitope in the Receptor Binding Domains of SARS-CoV-2 and SARS-CoV. Science 368, 630–633. doi:10.1126/science.abb7269
Keywords: COVID-19, main protease, sesame, natural compounds, molecular docking, molecular dynamics simulations, therapeutics
Citation: Kumar A, Mishra DC, Angadi UB, Yadav R, Rai A and Kumar D (2021) Inhibition Potencies of Phytochemicals Derived from Sesame Against SARS-CoV-2 Main Protease: A Molecular Docking and Simulation Study. Front. Chem. 9:744376. doi: 10.3389/fchem.2021.744376
Received: 20 July 2021; Accepted: 06 September 2021;
Published: 08 October 2021.
Edited by:
Sugunadevi Sakkiah, National Center for Toxicological Research (FDA), United StatesReviewed by:
Subhash Chandra, Kumaun University, IndiaMd Tabish Rehman, King Saud University, Saudi Arabia
Copyright © 2021 Kumar, Mishra, Angadi, Yadav, Rai and Kumar. This is an open-access article distributed under the terms of the Creative Commons Attribution License (CC BY). The use, distribution or reproduction in other forums is permitted, provided the original author(s) and the copyright owner(s) are credited and that the original publication in this journal is cited, in accordance with accepted academic practice. No use, distribution or reproduction is permitted which does not comply with these terms.
*Correspondence: Ulavappa Basavanneppa Angadi, ub.angadi@icar.gov.in